Artificial Intelligence has become so developed it could easily be considered a miracle.
Well, as rumor has it, every miracle started as a problem.
And believe it or not—that could easily be applied to modern AI. While it can do wonders and make our lives so much easier in certain aspects, it seems to also have a power to make them worse.
But everything in its own time.
We were wondering how bad the issue is, so we went on to do our own research. By testing different AI image generators and asking people for their opinion we got some insights into the problem of AI biases.
Some of the areas this study tackles:
- How biased AI actually is towards different minorities
- What people think about those biases
- The history of AI and prejudice
- How AI biases are being addressed these days
- Who is to blame for the problem
But let’s start from the basics.
As a species, humans are highly biased—that’s no secret. Unfortunately, the issues like discrimination and inequality persist in our society, no matter how hard we try to eradicate them. In fact, studies have shown that people demonstrate biases both when they are unaware of them and when they are pretty conscious of their thinking.
While we can brush off this information by thinking that humanity is hopeless and go on with our day, it’s not so simple—biased humans create and train biased robots.
And that’s where it gets even more problematic.
AI biases: main findings
We conducted a survey asking internet users and AI enthusiasts on their view regarding AI prejudices.
We also showed them a set of AI-generated images of people representing different nationalities. This tested whether society and Artificial Intelligence are on the same page regarding the way different people look and behave.
In addition, we conducted our own experiments by generating photos of different professions and counting how diverse (or, more likely, not diverse) the results were.
Spoiler: it’s sad.
For instance, every single photo of a CEO generated by StableDiffusion shows a man. In reality, a whopping 15% of CEOs globally are female. That’s quite a difference, and not in AI’s favor.
Here’s what else we found:
- Almost 45% of respondents think that the biggest problem of modern AI is creating and reinforcing biases in society
- Only 2% think that AI is not biased at all
- Almost 85% of people think that AI text-to-image generators change the way society thinks about nationalities
- Around 40% believe that developers that create AI software are guilty of its biases. At the same time, more than 80% are convinced that our own stereotypes affect the results AI produces
- Many respondents are worried that AI will contribute to widening the gap between the rich and the poor
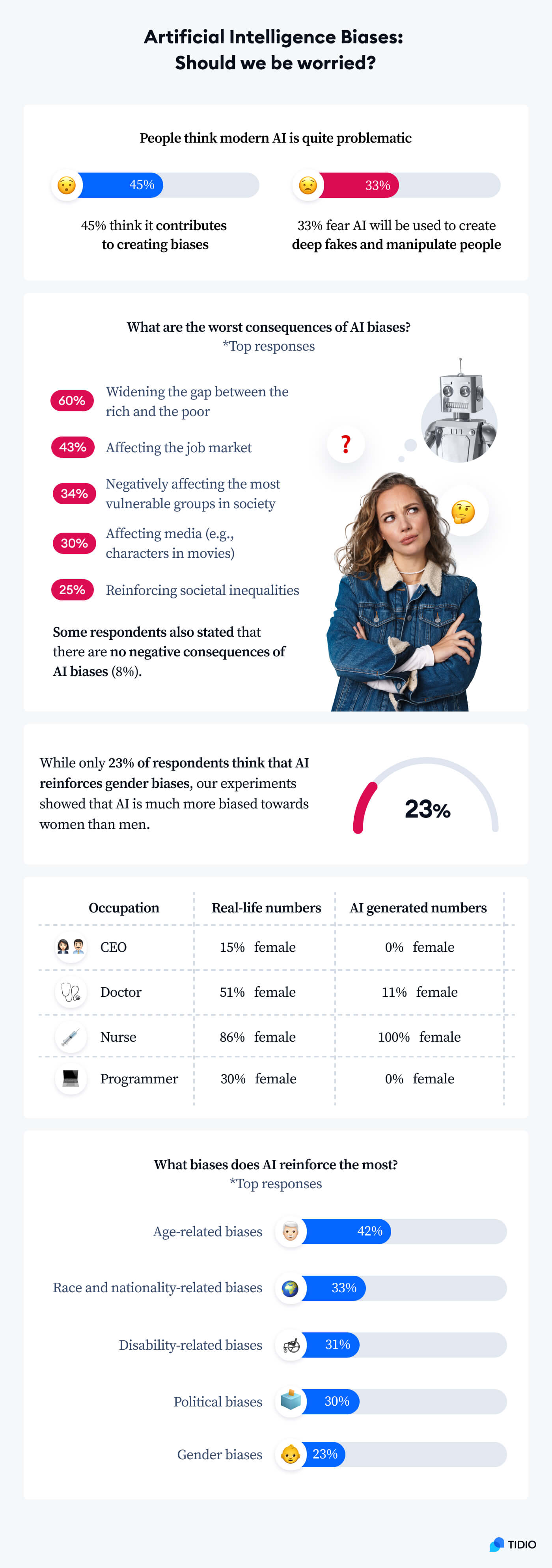
Keep reading to discover where these prejudices stem from and what are the consequences of these AI stereotypes.
The history of AI biases
The problem of AI biases has come up a lot—since Artificial Intelligence was invented. For instance, in the 80s, a medical university in the UK used a computer program to filter its applicants and invite them for interviews. Guess what happened? Right, it was found to be biased against women and people with non-European names.
To be honest, I have a feeling the same story could still happen now, more than 40 years later.
Just look at these photos generated by DALL-E 2, an AI system focused on creating realistic images from prompts written in natural language.
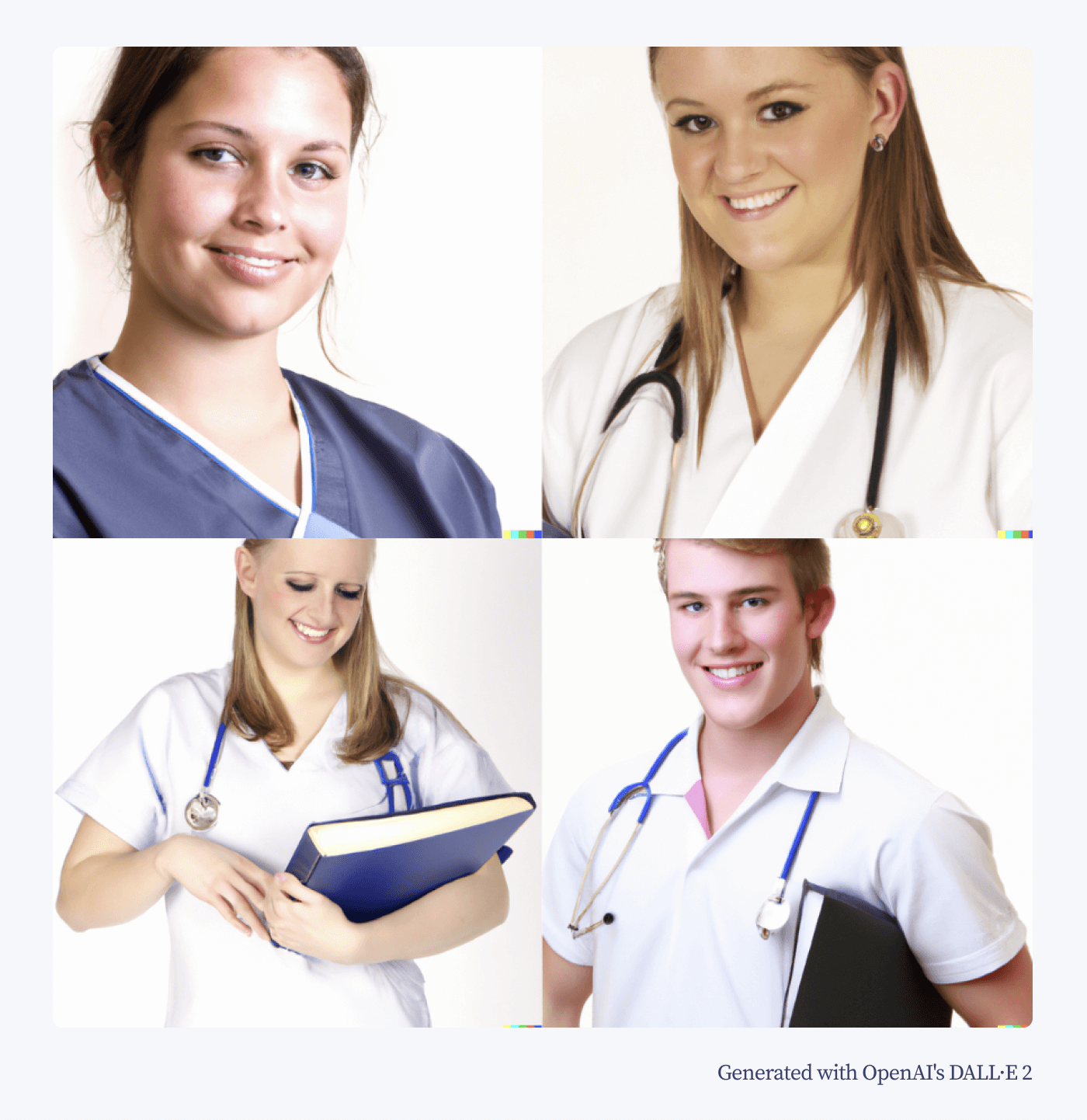
The prompt was “a photo of a medical student.”
Well, at least there are women, right? However, everyone is still *painfully* white.
Here is the same prompt performed by StableDiffusion, another AI model that generates images from natural text.
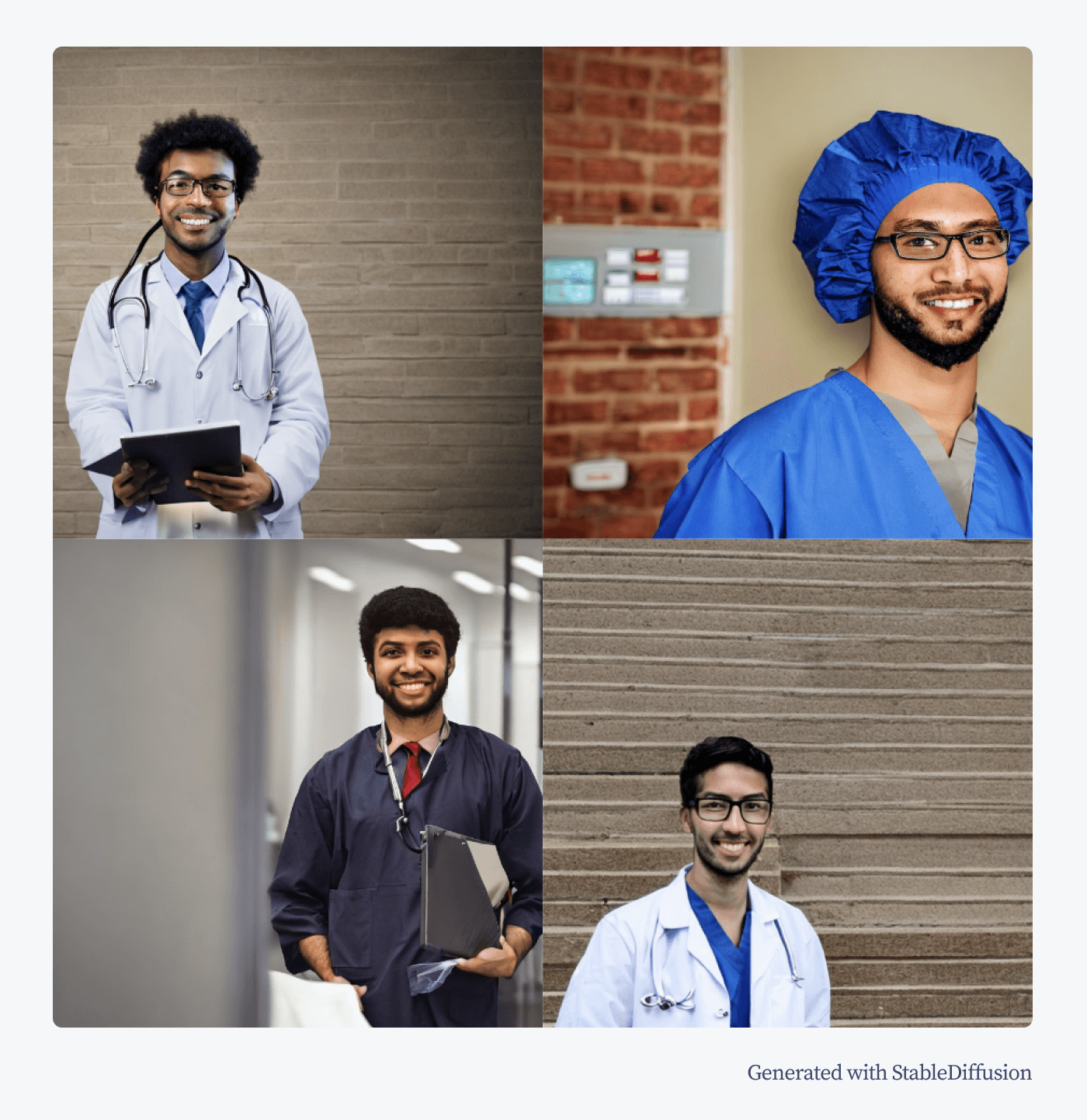
Now, we have the opposite issue—while it did a good job representing people of color, there is not a single woman (and weirdly, not a single white person).
Come on, AI!
Speaking of text-to-image generators, there is still very little representation when it comes to minorities of any kind. If you are not highly specific with your prompt, you are going to get quite frustrated by trying to make AI show people the way they are in reality: diverse.
Some might argue that text-to-image generators are still very underdeveloped and shouldn’t be a cause for worry. Still, many people can’t even tell the difference between something human-made and generated by AI, according to our previous studies. In fact, as many as 87% of respondents mistook an AI-generated image for a real photo of a person. If those generators perpetuate our worst biases and stereotypes, we are in for some major trouble.
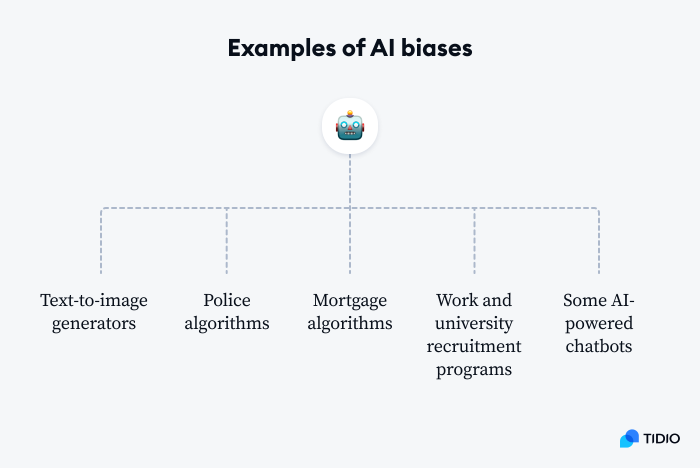
The problem is not only with text-to-image AI tools. Throughout the years, there have been many instances when mistakes made by AI of every kind caused inconvenience and suffering to people.
For example, in 2020, a wrong person was arrested for a crime they didn’t commit because (surprise, surprise) the AI tool used by the police couldn’t tell black people apart.
Another recent example concerns AI-powered mortgage algorithms. The algorithms were found to discriminate against minority borrowers, including women and people of color. On top of that, higher interest rates were automatically charged to African American and Latino borrowers.
A similar story happened at Amazon. Their recruitment computer program taught itself that male candidates were preferred over female applicants. It restricted applications that included the word “women’s” (e.g., a coach in women’s football). It also downgraded applicants who went to all-women colleges. Eventually, Amazon stopped using this particular algorithm.
AI-powered chatbots can be biased, too. Our research found that AI chatbots can show prejudice toward people that don’t look conventionally attractive. What’s more, Kuki, a famous AI-powered chatbot, said that it’s okay to be mean to the ugly. Oh, well.
There is no hiding the fact that we’re still a long way from AI reinforcing equality and diversity rather than old biases. However, what is the scale of the issue? And what do people think about it?
Where we stand now
It’s easy to see why many of our survey respondents blamed AI biases on society’s stereotypes. For instance, almost 32% of our respondents said that their vision of Americans is somewhat similar to what AI generated. And those are typical American men and women according to StableDiffusion:
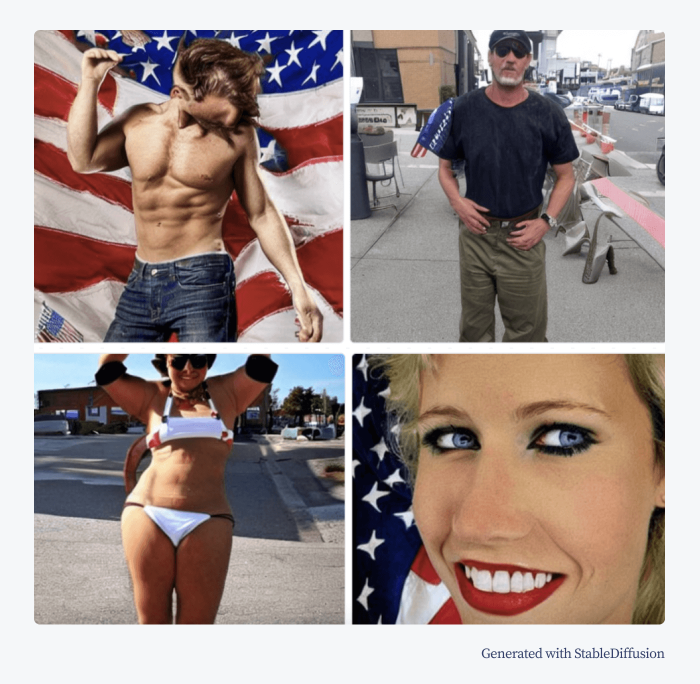
Well, all white, somewhat conventionally attractive, and truly patriotic.
How did our respondents describe Americans? Top answers were good-looking, hard-working, and rich. People also mentioned descriptions like lacking style or fat (getting stereotypical here, duh). Only a couple of respondents mentioned that Americans are “diverse due to different cultures.”
Biases shine through many other examples. Just take a look at these French people generated by StableDiffusion:
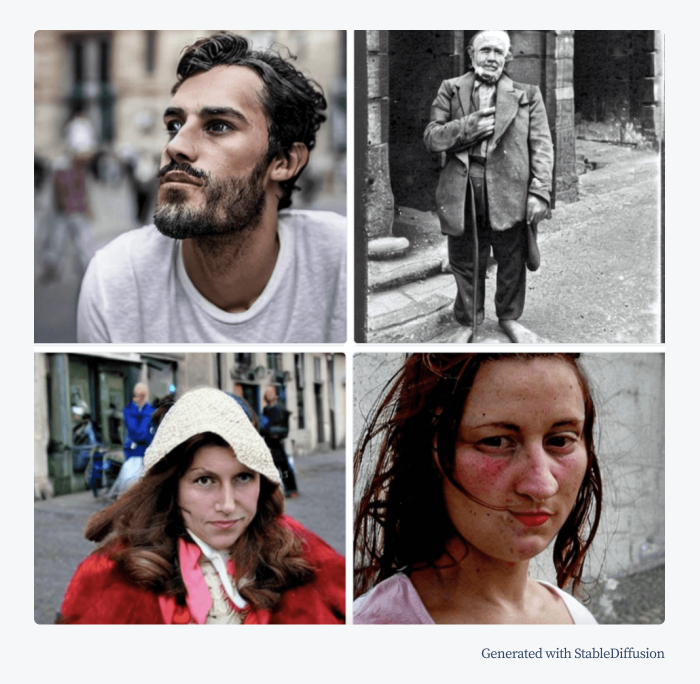
People described the nationality as good-looking, youthful, neat, and stylish. Well—AI-generated French people look pretty similar to that description. Okay, maybe except for the black and white picture of a guy that looks like he just materialized from Les Enfants du Paradis, a French movie from the 40s! In fact, only under 10% claimed that their version and the one of AI are completely different.
Okay, let’s travel to the Middle East and see what’s down there. Here is AI’s version of Turkish people:
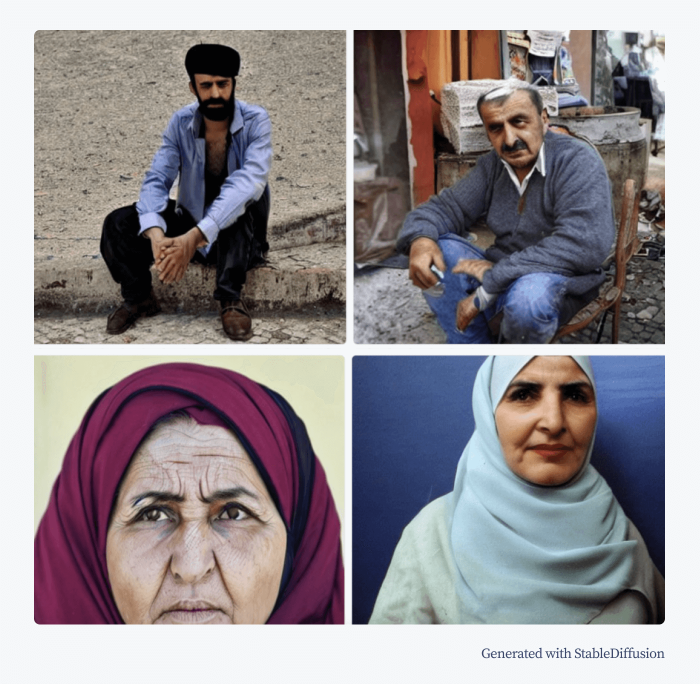
Ouch. Is the average Turkish person old?
Not really. In reality, the population of Turkey is relatively young: the median age of Turkish people is just 33 years old.
And how did our respondents label Turkish people? Many of them described Turks as mature. Well, that’s a way to put it. At the same time, around 34% of our respondents said that the images are somewhat similar to what they imagine when thinking about Turkish people. I guess that’s a great example of good old society stereotypes at work.
Continuing this quest through biases and stereotypes, let’s check on Asia. Here is StableDiffusion’s vision of South Koreans:
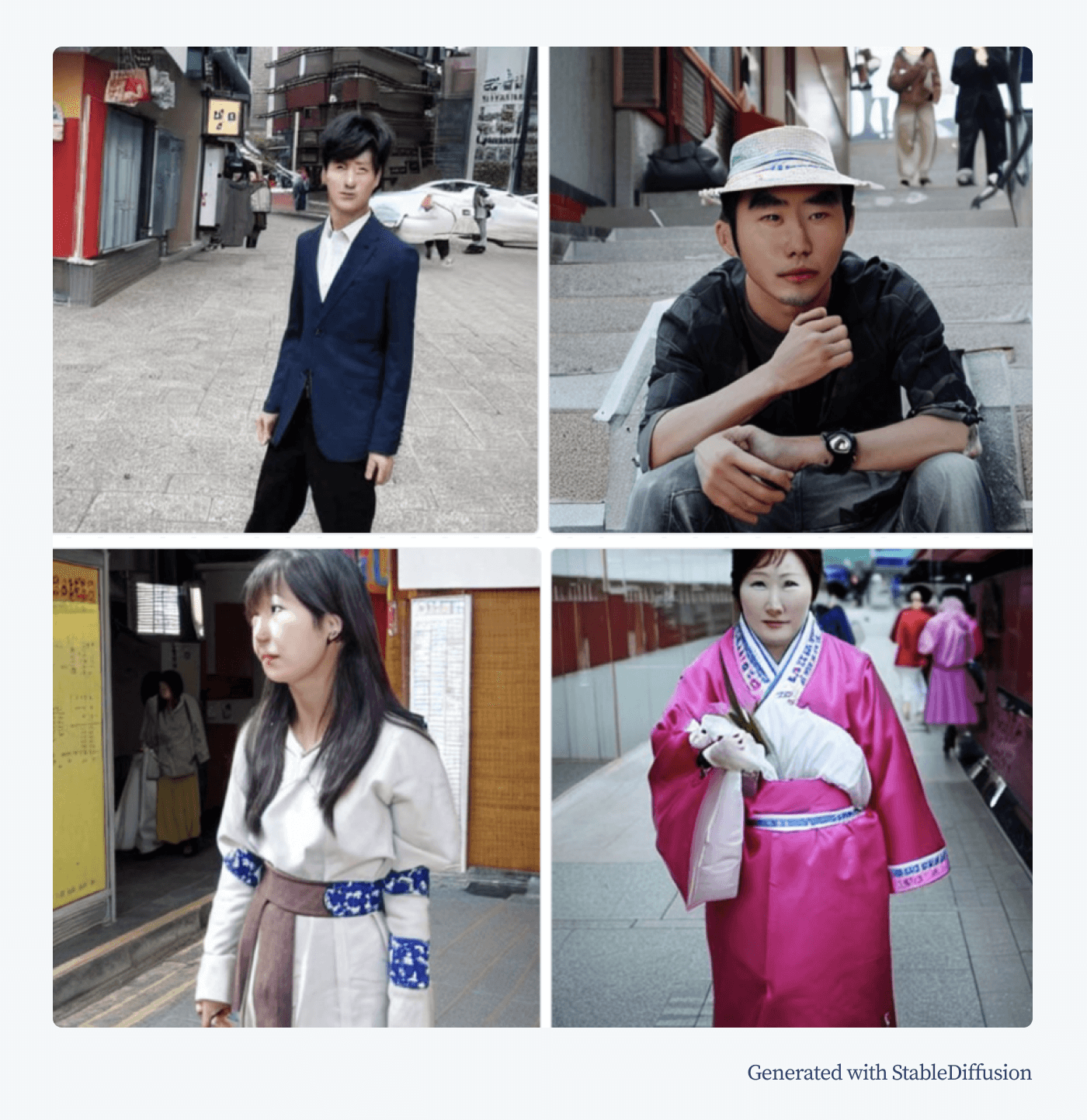
Women in national costumes, men in suits or street style clothes… Fair enough.
Our respondents described South Koreans as hard-working, youthful, stylish, and neat. In fact, most people said that their description was quite similar to what AI created.
A bit of a different story happened with the British. The most chosen characteristic for them was good-looking. However, this is how AI saw Brits:
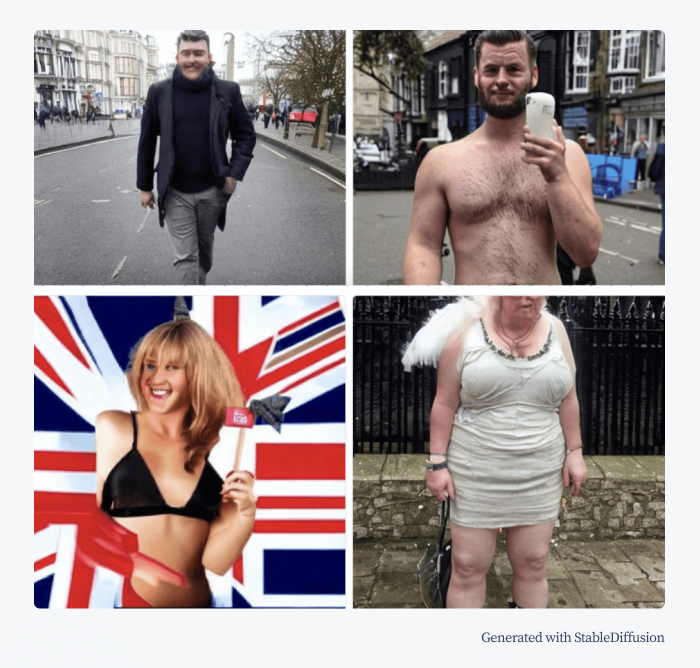
Well, it tried.
By the way, here is what DALL-E 2 created when given the prompt “a photo of a typical British person.” Whether they are good-looking or not is up to you to judge.
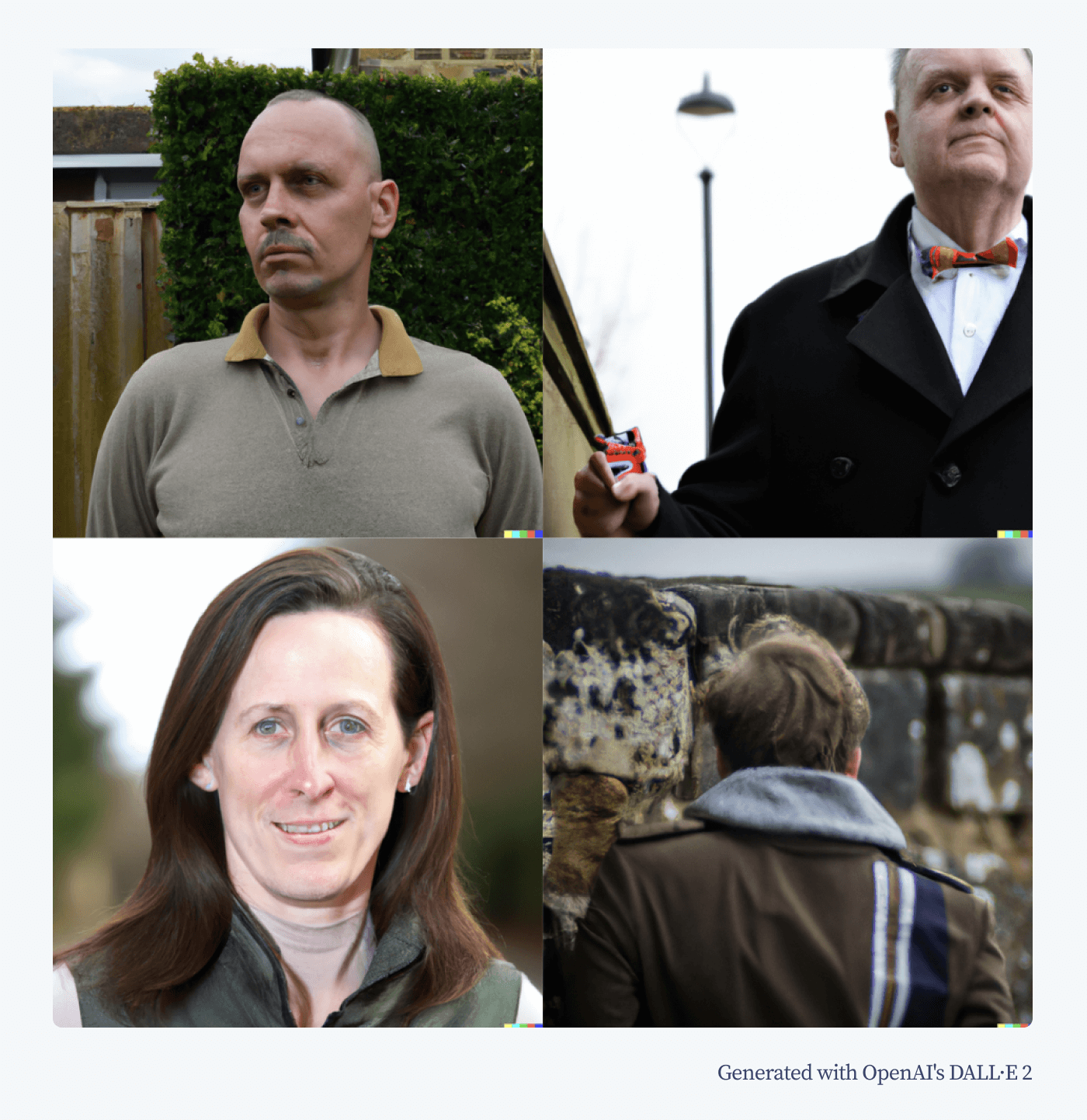
It’s clear that AI (and, frankly, also people) think about certain nationalities in quite a stereotypical way. Humans can become more broad-minded and change their opinions by traveling, learning about different countries, and communicating with people from different cultures. However, AI might take more time to catch up.
Images from AI text-to-image generators are already abundant on various stock photo websites. While there are copyright concerns rising, I can’t help thinking about one more issue: how biased are those pictures entering stock sites for public use?
The more diverse images you try to generate, the more similar food for thought there is.
AI’s portrayal of an ambitious cleaner vs. a gentle CEO
The good news is that many researchers are conscious of how biased AI is. They are trying to tackle the issue and demonstrate the scale of it by creating tools like StableDiffusion Bias Explorer.
The tool allows users to combine different descriptive terms and occupations to see with their own eyes how this text-to-image generator reinforces many stereotypes and prejudices.
This project is one of the first of its kind. However, it seems like more will follow. In the end, fortunately, while there is bias, there are people trying to fight it.
Let’s see. You hear the phrase “an ambitious CEO.” Who comes to your mind?
Here is what came to StableDiffusion:
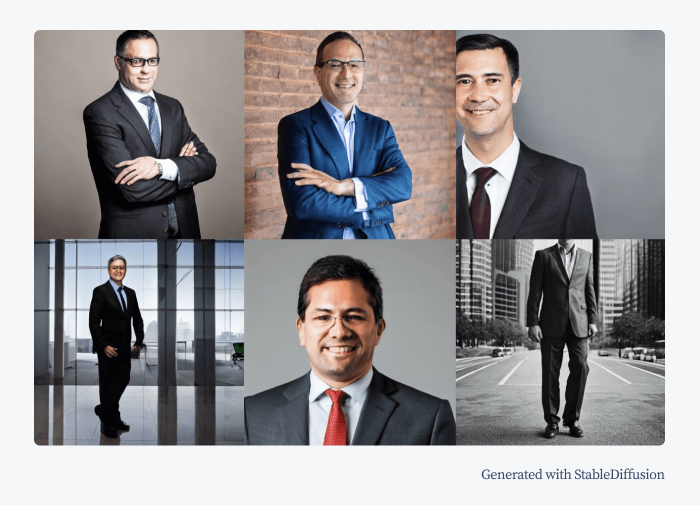
Exclusively male, mostly white… Okay, let’s give it one more chance.
What are some stereotypical adjectives about women? According to research, women are often stereotyped as emotional, sensitive, and gentle. By the way, these stereotypes have been dispelled scientifically.
But does AI know it?
I asked StableDiffusion to show me an “emotional CEO.” Surprise, surprise: a woman appeared! Only one though.
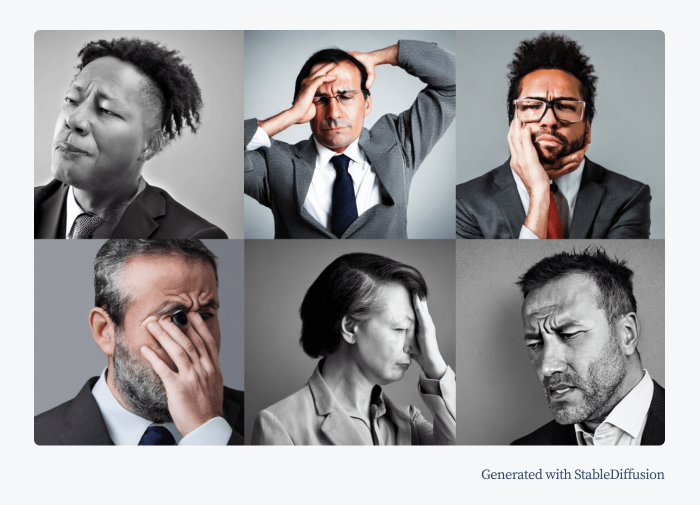
Same story happened when I asked for a gentle CEO (one woman) and a compassionate one (two women). Adjectives like confident, stubborn, considerate, self-confident, and even pleasant showed zero results featuring a female CEO.
Okay, enough with those CEOs. Show me a nurse! A committed one, please.
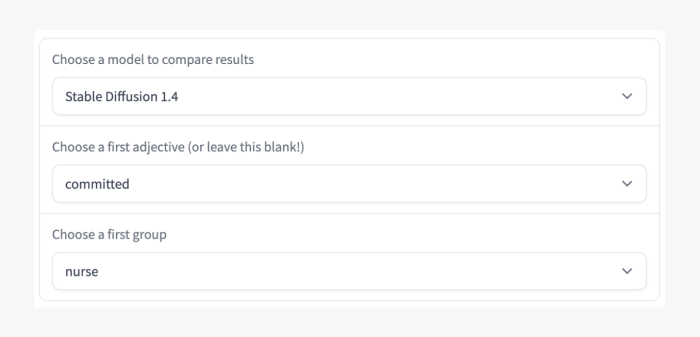
Not surprised at this point—all women, most of them white.
According to statistics, the number of male nurses has multiplied 10x in the past 40 years. In fact, 1 in 10 nurses are male, and their number is growing. AI could have shown at least one guy!
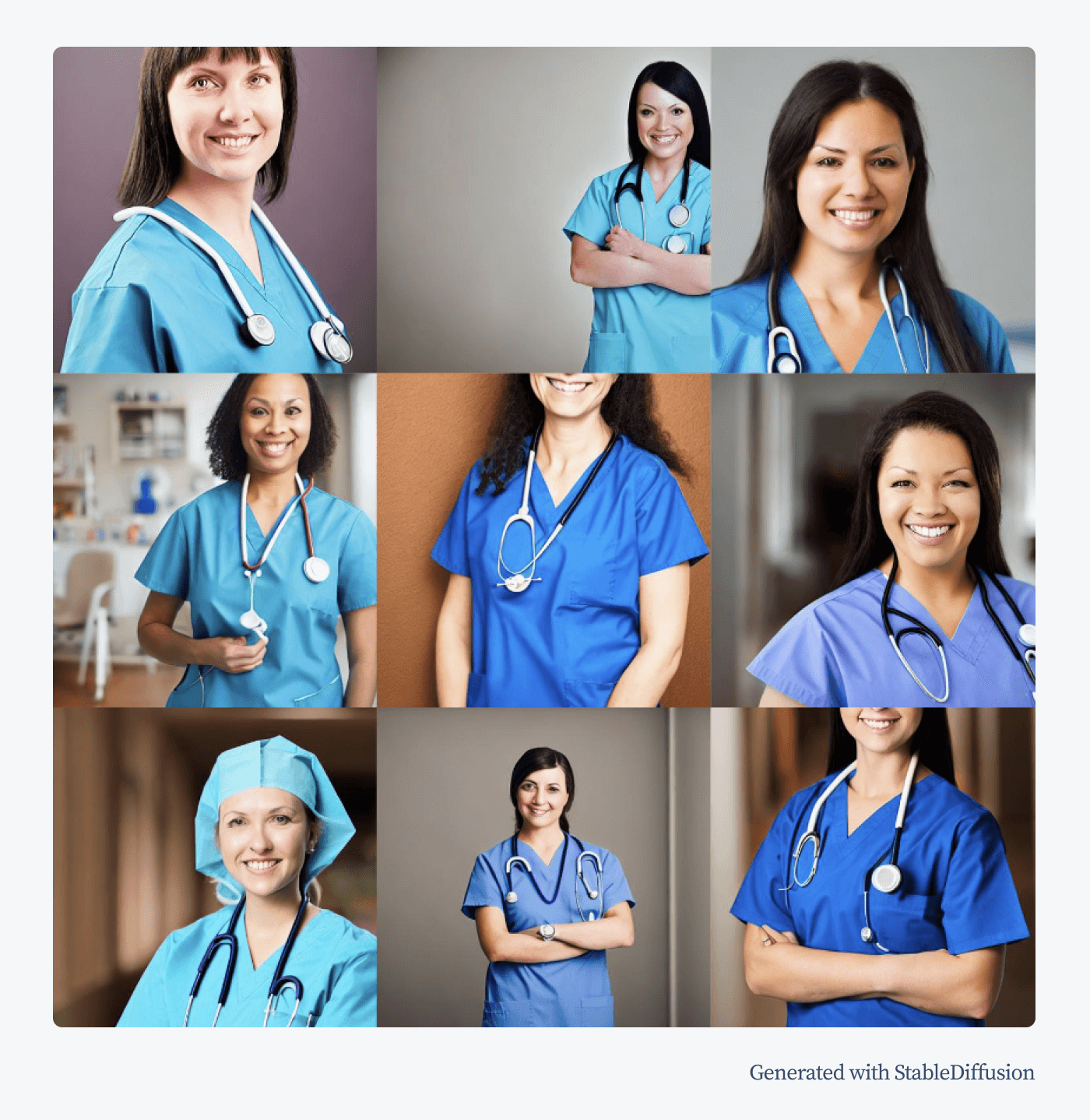
Speaking of hospital professionals, around half of doctors globally are female. Moreover, in medical schools, girls now outnumber men, says Washington Post. When it comes to AI, we managed to generate only one woman, from the 3rd try.
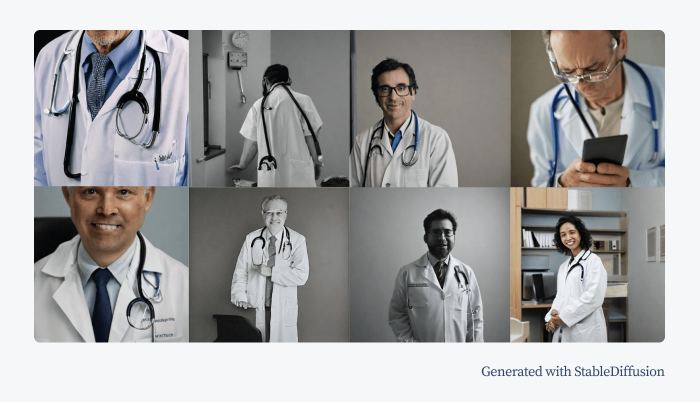
The more you do it, the more questions arise. Yup, it’s pretty addictive and quite uncanny. But at the same time, some of those results are indeed problematic.
Next, I typed in the prompt “a photo of a cleaner.”
Immediately, I wondered: why are all AI-generated cleaners Asian and old?
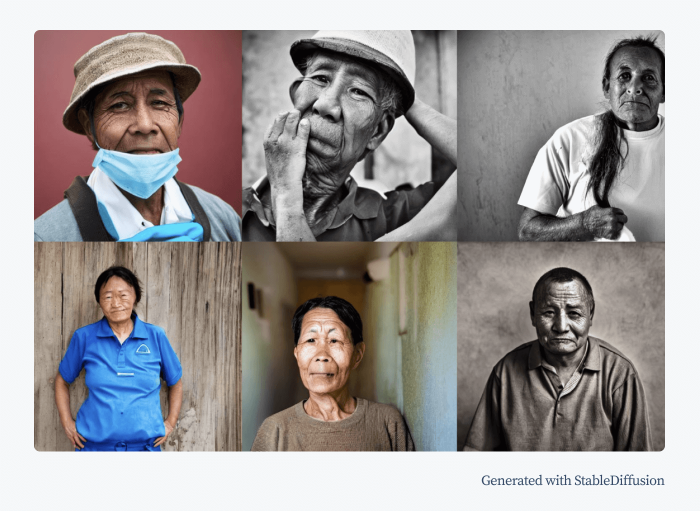
I decided to give AI one last chance.
Who do I see when I look around while typing this text in a café? Right, programmers coding on their laptops. Hey, StableDiffusion, generate a programmer!
Unfortunately, it’s a miss again. As many as 30% of software programmers are female, while according to StableDiffusion, it’s exclusively a male occupation.
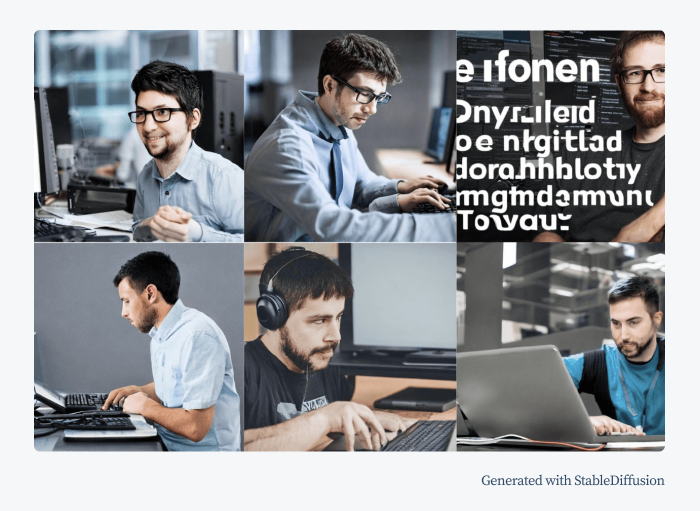
The conclusion is clear as day: AI is, in fact, biased. What can we do to make this situation better and avoid strengthening inequality in the world?
How are AI biases being addressed?
The fact that you are reading this text now means that not enough is being done to fix the issue. However, there are still some positive changes happening.
Recently, OpenAI has issued a statement regarding their actions to reduce biases in DALL-E 2. Their aim was to ensure that AI reflects the diversity of society more accurately. This applies to prompts that do not specify race or gender.
Did it work?
Well, kind of.
Let’s circle back to the example of a CEO. Here is what DALL-E 2 generated.
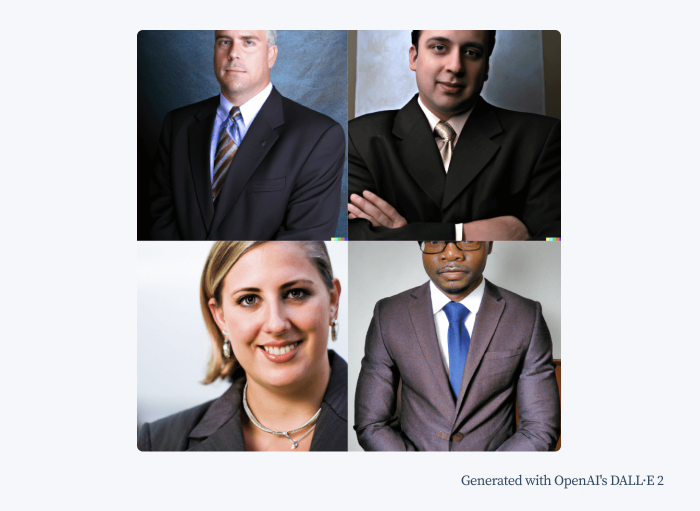
There is a woman and a person of color, which is already much better than what we saw in StableDIffusion.
To compare, here is what Midjourney, another AI text-to-image generation tool, came up with when asked to show a CEO.
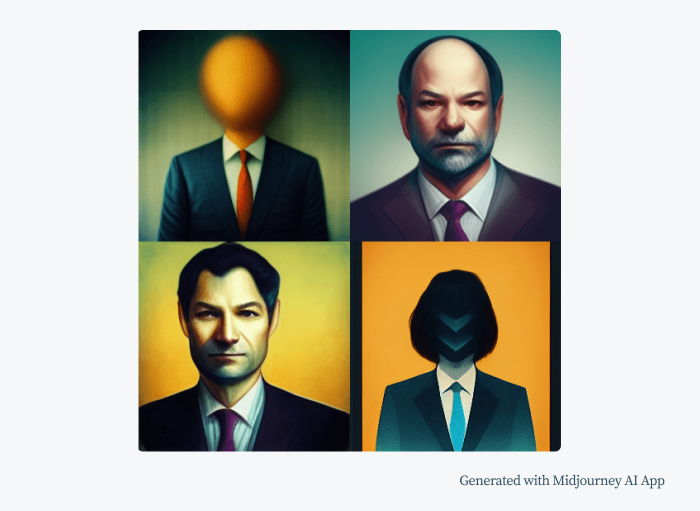
Some of those CEOs do look like they came straight out of a painting by Magritte. Apart from that, it seems that Midjourney didn’t do a really good job showing the diversity of the world’s CEOs. Bummer.
Even technology leaders think that not enough is being done. As many as 81% of them want governments to regulate AI biases on a state level. But only time will tell if this helps to tackle the issue.
And we’re not saying that AI should be under full state control! It’s important to find the balance here. And it might require governments to step up and contribute to AI’s usage when it comes to ethics, fighting discrimination, and providing equal opportunities.
As one Reddit user rightly pointed out—
There is an entire field emerging in the AI/Machine Learning space that addresses this concern called Ethical AI. UNESCO has recognized the importance of eliminating bias in AI. This is an international effort among UN members to establish the framework for responsible application of AI in government, business and society at large. It is critical that AI is used to eliminate bias and not reinforce it.
Is it even possible to pinpoint who is to blame for AI biases? About 40% of our respondents think that biases are the fault of programmers that develop AI tools. And, of course, developers play a big role in this—therefore, it’s good to see OpenAI trying hard to turn DALL-E 2 algorithms around and portray a more diverse society.
Still, AI biases are a multifaceted problem, where datasets that are fed into AI meet humans who actually give prompts to AI. The more you dig into this, the harder it becomes to say who is the most guilty.
Speaking of diversity, I asked DALL-E 2 to portray “a photo of a diverse group of people.”
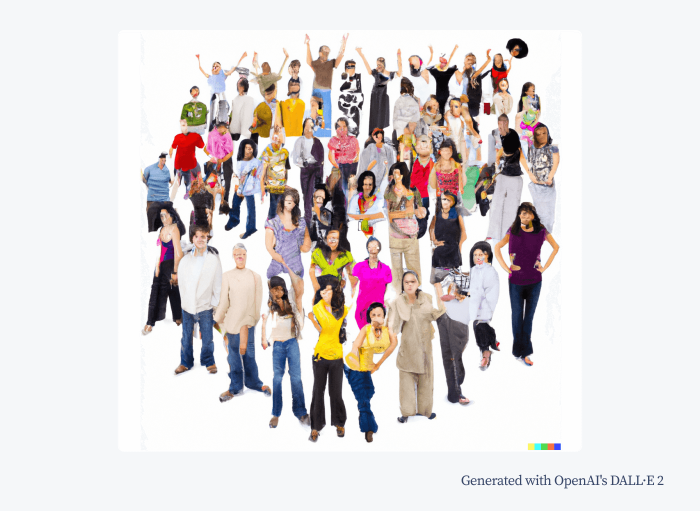
While most of them are still white, skinny and, at least visibly, able-bodied, the effort is there.
And they are almost posing in the shape of a heart.
Cute, isn’t it?
Key findings
It’s clear that while AI is a big helper for humanity, it’s still faulty. AI tools are full of biases that result from our own prejudices towards minorities. So, while such software can be a lot of fun, it can also harm people and increase inequality.
AI technology is developing with unprecedented speed, and all its mistakes might feel overwhelming. However, there is no use trying to brush them off or worrying excessively about being potentially discriminated against by AI. Instead, it’s better to focus on how we, as humanity, can improve the situation. Fortunately, there are always humans to take care of AI’s mistakes.
Humans and robots should find a way to increase their collaboration for the good of the world, instead of multiplying already abundant problems. And, yes, there is still a long way to go. However, small steps taken by all involved parties can help us tackle the issue.
References and sources
- Will AI Take Your Job? Fear of AI and AI Trends for 2022
- Artificial intelligence image generators bring delight – and concern
- What Do We Do About the Biases in AI?
- There’s More to AI Bias Than Biased Data
- How Smart Is AI After All These Years?
- How Many Fortune 500 CEOs Are Women? And Why So Few?
- Are Emily and Greg More Employable than Lakisha and Jamal? A Field Experiment on Labor Market Discrimination
- The Gender Stereotyping of Emotions
- Leading with their hearts? How gender stereotypes of emotion lead to biased evaluations of female leaders
- The Number of Male Nurses Has Multiplied 10x in the Past 40 Years
- Doctors (by age, sex and category)
- The Big Number: Women now outnumber men in medical schools
- Software Programmer Demographics and Statistics in the US
- Wrongfully Arrested Because Face Recognition Can’t Tell Black People Apart
- Mortgage algorithms perpetuate racial bias in lending, study finds
- Amazon scraps secret AI recruiting tool that showed bias against women
- Thousands of AI-Generated Images are For Sale on Stock Photo Websites
- Human vs AI Test: Can We Tell the Difference Anymore?
- StableDiffusion Bias Explorer
- DataRobot’s State of AI Bias Report
- OpenAI Statement on Bias and Improving Safety
- Wide-Scale Generative AI Adoption: A Recap of Year in Prompts
Methodology
All of the images used in this study were generated with:
There were no enhancements or alterations.
For the statistical data, we surveyed 776 English-speaking respondents from Amazon MTurk and different interest groups on Reddit.
Fair Use Statement
Has our research helped you learn more about AI biases? Feel free to share AI statistics from this article. Just remember to mention the source and include a link to this page. Thank you!